Abstract: Tumors employ complex, multi-scale cellular and molecular interactions that evolve over the course of therapeutic response. The changes in these pathways enables tumors to overcome therapeutic regimens, and ultimately acquire resistance. New molecular profiling technologies, including notably single cell technologies, provide an unprecedented opportunity to characterize these molecular relationships. However, interpreting the specific cellular and molecular pathways in therapeutic response requires complementary computational analysis methods. We developed an unsupervised learning method, CoGAPS, that employs Bayesian non-negative matrix factorization to disentangle distinct biological processes from high-throughput molecular data. Notably, this algorithm discovers dynamic compensatory signaling in acquired therapeutic resistance from time course bulk RNA-seq data and novel NK cell activation in anti-CTLA4 response from post-treatment scRNA-seq data. To further demonstrate that the inferred pathways are biological rather than computational artifacts, we developed a complementary transfer learning method to relate learned patterns between datasets. We demonstrate that this approach identifies robust molecular processes between model systems and human tumors and enables multi-platform data integration to delineate the drivers of therapeutic response and resistance.
Bioinfo4Women seminars / Virtual BSC RS/BSC Life Session
Enter the matrix: modeling tumor cell and immune cell interactions at the single-cell resolution
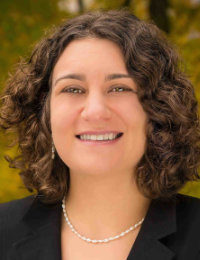
Speaker:
Associate Professor of Oncology, Assistant Director of @HopkinsRPQS SKCCC, Johns Hopkins